Abstract:
Automating leaf detection and physical leaf sample collection using Internet of Things (IoT) technologies is a crucial task in precision agriculture. In this paper, we present a deep learning-based approach for detecting and segmenting crop leaves for robotic physical sampling. We discuss a method for generating a physical dataset of agricultural crops. Our proposed pipeline incorporates using an RGB-D camera for dataset collection, fusing the depth frame along with RGB images to train Mask R-CNN and YOLOv5 models. We also propose our novel leaf pose estimating algorithm for physical sampling and maximizing leaf sample area while using a robotic arm integrated to the P-AgBot platform. The proposed approach has been experimentally validated on corn and sorghum, in both indoor and outdoor environments. Our method has achieved a best-case detection rate of 90.6%, a 9% smaller error compared to our previous method, and approximately 80% smaller error compared to other state-of-the-art methods in estimating the leaf position.
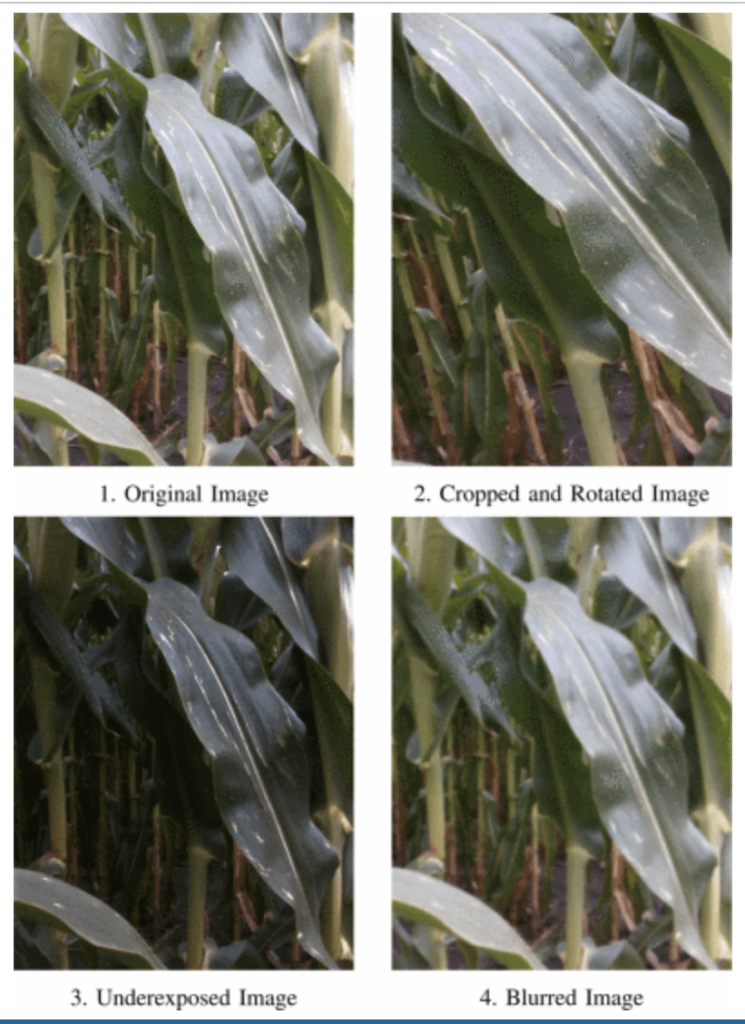
Published in: 2023 IEEE/RSJ International Conference on Intelligent Robots and Systems (IROS)